Sano’s Projects
At Sano Centre for Computational Medicine, we are proud to showcase the diverse and showcase diverse set of projects that our teams and individual researchers are undertaking, thanks to the support from a variety of funding sources including European and Polish grants. Each project reflects our commitment to advancing the field of Computational Medicine through innovative research, interdisciplinary collaboration, and the application of advanced computing technologies to solve complex medical challenges.
Here, you will find detailed insights into the aims, and impacts of our ongoing and completed projects.
These initiatives not only contribute to scientific knowledge but also aim to enhance healthcare outcomes, demonstrating our dedication to improving patient care through scientific excellence.
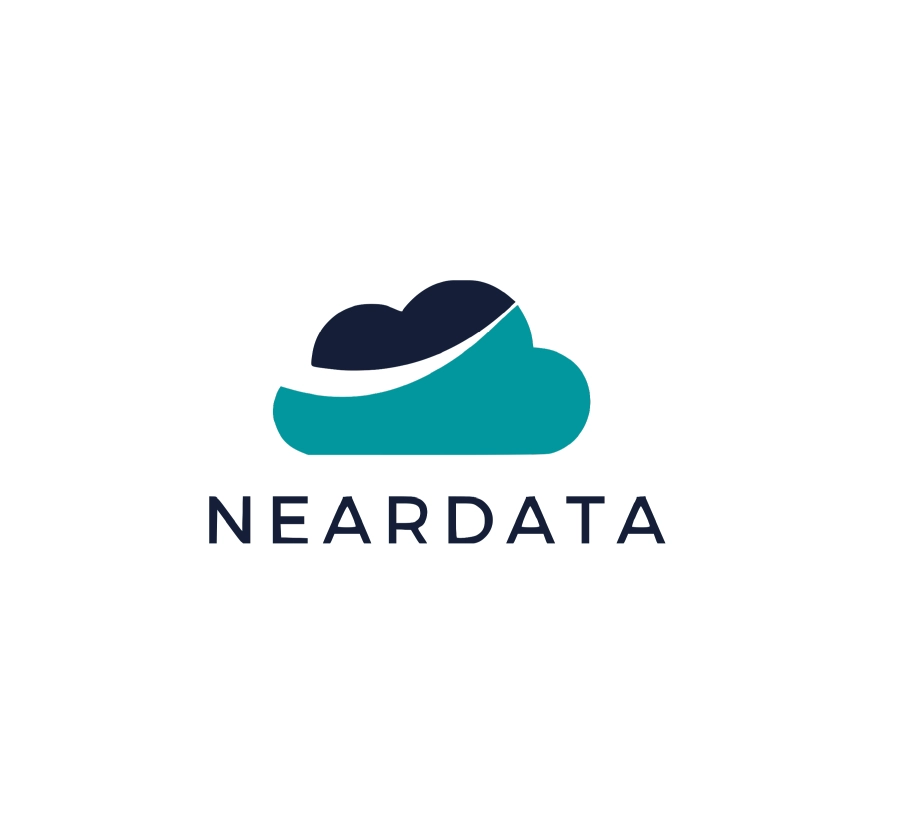
NEARDATA
The goal of NEARDATA is to create an extreme data infrastructure mediating data flows between Object Storage and Data Analytics platforms across the Compute Continuum. Novel XtremeDataHub platform is an intermediary data service that intercepts and optimises data flows (S3 API, stream APIs) with high performance near-data connectors (Cloud/Edge).
Sano Team is responsible for:
- Developing a pipeline for building transcriptomics atlas of selected tissues/diseases, with the use of HPC and Cloud technologies;
- Federated Learning framework – a set of tools for running Federated Learning experiments on large scale genomics data.
NEARDATA project website: https://neardata.eu/
Duration: 01 January 2023 – 31 December 2025
Partners/ List of collaborators:
- Universitat Rovira i Virgili (Spain) – Coordinator
- Barcelona Supercomputing Center (Spain)
- Technische Universität Dresden (Germany)
- Deutsches Krebsforschungszentrum Heidelberg (Germany)
- European Molecular Biology Laboratory (Germany)
- EMC Information Systems International Unlimited Company (Ireland)
- KIO Networks España SA (Spain)
- Sano – Centre for Computational Medicine (Poland)
- Scontain GMBH (Germany)
- UK Health Security Agency (United Kingdom)
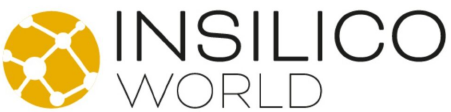
ISW (In Silico World)
The In Silico World project aims at accelerating the uptake of modelling and simulation technologies used for the development and regulatory assessment of medicines and medical devices, by lowering seven identified barriers: development, validation, accreditation, optimisation, exploitation, information, and training.
This initiative employs computer models that leverage experimental data for hypothesis testing and outcome prediction.
Work package:
Scalability and efficient computing (WP5 led by Sano)
This package focuses on creating a sophisticated, user-friendly simulation platform that ensures the repeatability, replicability, and reproducibility of simulation outcomes. It also aims to facilitate efficient access and utilization of computational and storage capacities, both locally and within major European e-infrastructures like PRACE, EOSC, Eudat, and upcoming EuroHPC initiatives.
ISW (In Silico World) project website: https://insilico.world/
Duration: 1 January 2021 –31 December 2024
Partners/ List of collaborators:
- Alma Mater Studiorum – Università di Bologna (UNIBO) – Coordinator
- Universiteit van Amsterdam (UvA)
- Technische Universiteit Eindhoven (TU/e)
- Università degli Studi di Catania (UNICT)
- Virtual Physiological Human Institute for Integrative
- Biomedical Research VZW (VPHi)
- Katholieke Universiteit Leuven (KU Leuven)
- Insilicotrials Technologies SRL (IST)
- Universite de Liege (ULIEGE)
- Erasmus Universitair Medisch Centrum Rotterdam (EMC),
- Budapesti Muszaki es Gazdasagtudomanyi Egyetem (BME)
- Din Deutsches Institut Fuer Normung E.V. (DIN)
- Mimesis SRL (MIM)
- Rsscan International NV (RSS)
- Sano – Centre for Computational Medicine (Sano)
EU
Teaming for Excellence (2019 – 2026) Maciej Malawski (Dyrektor)
InSilicoWorld ISW (2021 – 2024) Marian Bubak(Dyrektor ds. naukowych Sano)
NearData (2023 – 2025) Maciej Malawski (Extreme-scale Data and Computing)
GEMINI (2023 – 2029) Marian Bubak(Dyrektor ds. naukowych Sano)
NCN
WEAVE (2024 – 2027) Tomasz Kościółek (Structural and Functional Genomics)
SONATA (2024 – 2027) Barbara Pucelik (Structural and Functional Genomics)
PRELUDIUM (2025 – 2027) Joan Roget Falco (Computer Vision)
PRELUDIUM (2024 – 2025) Magdalena Otta (Extreme-scale Data and Computing)
PRELUDIUM (2024 – 2027) Michał Grzeszczyk (Health Informatics)
NCBiR
.
Commercial
CACTUS