138. Emerging Technologies in Neuroimaging and AI for Enhanced Brain Pattern Analysis and Diagnosis
Rosmary Blanco – PhD Student, Computer Vision (Brain&More), Sano Centre for Computational Medicine, Krakow, PL
Abstract
Neurotechnology is an interdisciplinary field merging neuroscience, engineering, and computer science to study and control the nervous system. Wearable EEG devices capture synchronized oscillatory brain patterns through portable, user-friendly equipment [1]. The emerging perspective of neurodegenerative diseases as ‘oscillopathies’ underscores the utility of this technology in monitoring clinical conditions [2].
EEG presents several challenges, including a low signal-to-noise ratio, non-stationarity, non-linearity, volume conduction phenomena, and variability. Wearable EEG technology adds further complexities: more artifacts, fewer sensors, and lack of control in lab settings and standardization. To address these issues, state-of-the-art preprocessing techniques, advanced signal processing, and efficient feature engineering ML-based approaches have been proposed to improve data quality, handle complexity, and enhance interpretability [3].
In this research, multi-modal approaches and effective data modeling were employed to capture the complex spatiotemporal patterns of brain activity. These methods extend beyond traditional EEG approaches, enabling the development of innovative solutions for diagnosing, monitoring, and guiding interventions in neurodegenerative diseases.
First, the integration of EEG and fNIRS data, using a multilayer network model, was explored since these technologies can potentially be used in real-world applications [4]. Then, by integrating diffusion MRI data, we investigated the brain structure-function relationship through graph signal processing. This approach could detect changes in brain activity in the presence of disease-specific structural damage [5].
Finally, a multi-modal approach, integrating cognitive, behavioral, and EEG data, was employed to enhance the performance of an ML model in distinguishing mild cognitive impairment (MCI) from healthy controls. This approach was implemented in a novel wearable EEG-based framework developed as a tool for assessing cognitive impairment.
Ongoing research aims to investigate whether selected EEG features can track normal aging and distinguish it from MCI, potentially uncovering digital biomarkers of dementia.
[1] Zhao, H., Cao, J., Xie, J., Liao, W. H., Lei, Y., Cao, H., … & Bowen, C. (2023). Wearable sensors and features for diagnosis of neurodegenerative diseases: A systematic review. Digital Health, 9, 20552076231173569.
[2] Nimmrich, V., Draguhn, A., & Axmacher, N. (2015). Neuronal network oscillations in neurodegenerative diseases. Neuromolecular medicine, 17, 270-284.
[3] Amer, N. S., & Belhaouari, S. B. (2023). EEG Signal Processing for Medical Diagnosis, Healthcare, and Monitoring: A Comprehensive Review. IEEE Access.
[4] Blanco, R., Koba, C., & Crimi, A. (2023). Investigating the interaction between EEG and fNIRS: a multimodal network analysis of brain connectivity. bioRxiv, 2023-11.
[5] Blanco, R., Preti, M. G., Koba, C., Van De Ville, D., & Crimi, A. (2024). Structure-Function Relationship in Electrical and Hemodynamic Brain Networks: Insights from EEG and fNIRS during Rest and Task States. bioRxiv, 2024-04.
About the author
Rosmary holds a Master of Science in Medical and Molecular Biotechnology from the University of Verona, Italy. Her background is enriched by years of experience in clinical settings in the fields of neurology, neurosurgery, and neurorehabilitation. Her research has been dedicated to complex electrophysiological signal processing, focusing particularly on EEG source imaging and network analysis in epilepsy. At Sano, she is actively involved in research in network neuroscience to study the large-scale brain networks underlying neuronal communication, cognition, and human behavior, as well as in neurotechnology to explore their applications in real-world scenarios to aid decision-making and support diagnosis.
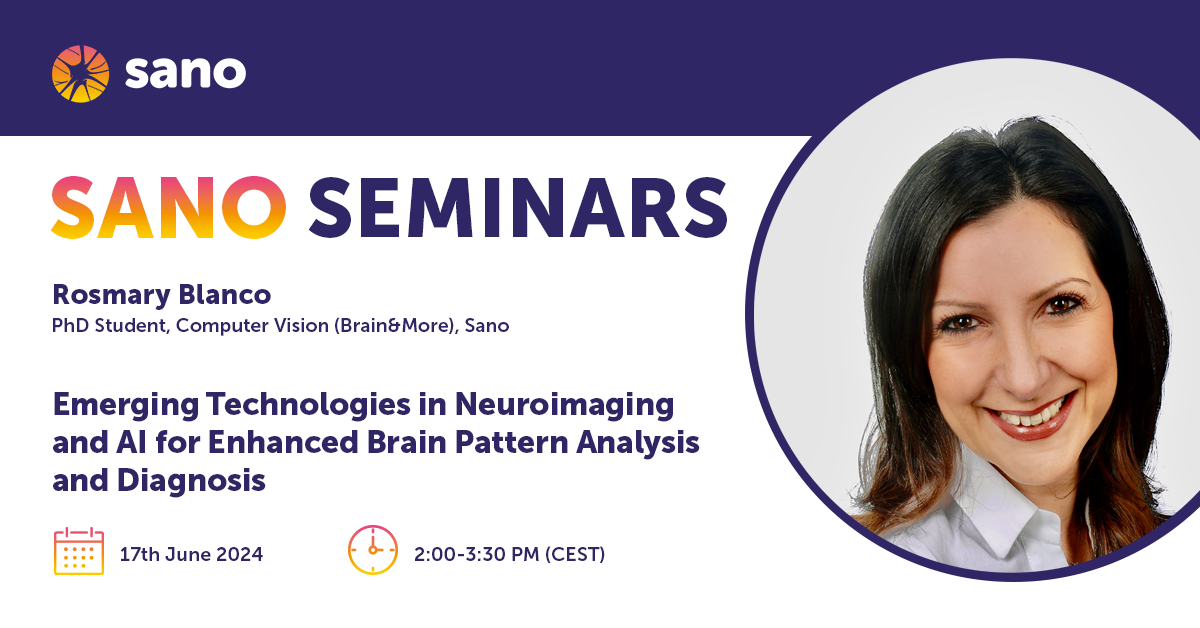