142. Robotic Assisted UltraSound (RAUS)
Michał Motak, PhD Student, Health Informatics, Sano Centre for Computational Medicine, Krakow, Poland
Abstract:
Ultrasound (US) is one of the most widely used modalities for clinical intervention and diagnosis providing non-invasive, radiation-free, and real-time images [1]. However, this examination depends on highly experienced sonographers to achieve high-quality US images. Low- and middle-income countries are most affected by this limitation [2].
Robotic Ultrasound Systems (RUS) are being developed to provide higher quality, faster and less operator dependent ultrasound examinations. RUS has wide applications such as teleoperation assistance, collaborative assistance for medical personnel, or semi and fully autonomous systems [3].
The capabilities of Robotic Assisted Ultrasound (RAUS) are being enhanced by rapidly growing technologies such as deep learning and virtual reality. Reinforcement learning (RL) is utilized to train RAUS in realistic virtual environments to perform automated ultrasound procedures. Path planning, proper probe orientation and force control are the main aspects of the training [4].
The aim of the research is to improve the standard approach to the training of robotic systems using deep learning methods and real-time analysis of acquired ultrasound images.
[1] Jiang, Zhongliang & Salcudeanb, Septimiu & Navab, Nassir. (2023). Robotic Ultrasound Imaging: State-of-the-Art and Future Perspectives.
[2] Ginsburg, A.S., Liddy, Z., Khazaneh, P.T. et al. A survey of barriers and facilitators to ultrasound use in low- and middle-income countries. Sci Rep 13, 3322 (2023). https://doi.org/10.1038/s41598-023-30454-w
[3] von Haxthausen, F., Böttger, S., Wulff, D. et al. Medical Robotics for Ultrasound Imaging: Current Systems and Future Trends. Curr Robot Rep 2, 55–71 (2021). https://doi.org/10.1007/s43154-020-00037-y
[4] H. Hase et al., “Ultrasound-Guided Robotic Navigation with Deep Reinforcement Learning,” 2020 IEEE/RSJ International Conference on Intelligent Robots and Systems (IROS), Las Vegas, NV, USA, 2020, pp. 5534-5541, doi: 10.1109/IROS45743.2020.9340913.
About the author:
Michał graduated from AGH University of Science and Technology with a Master’s degree in Biomedical Engineering. His area of research during university was segmentation and generalization problem of cardiac MRI data. At Sano, he is focusing on deep learning in robotic systems for automated ultrasound examination and fetal growth assessment.
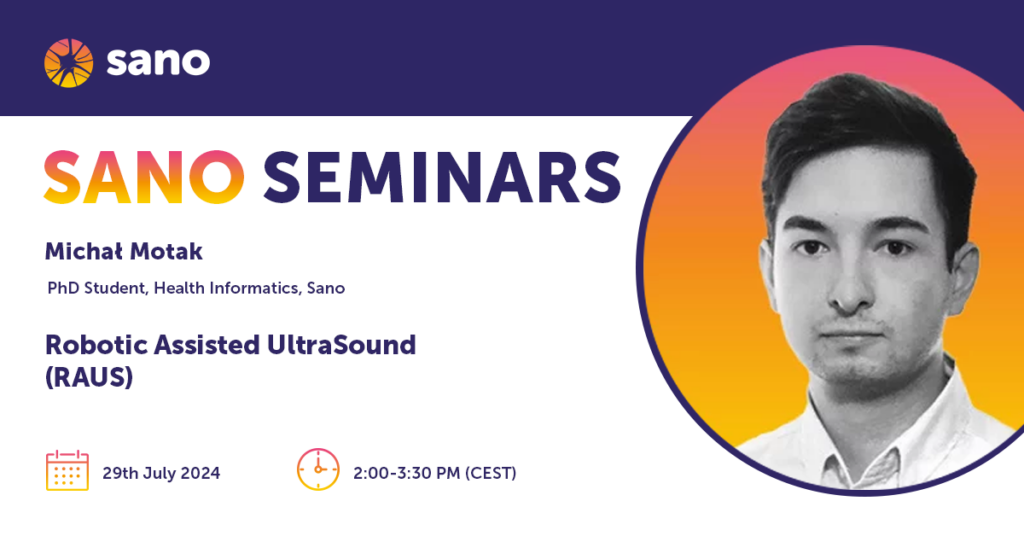