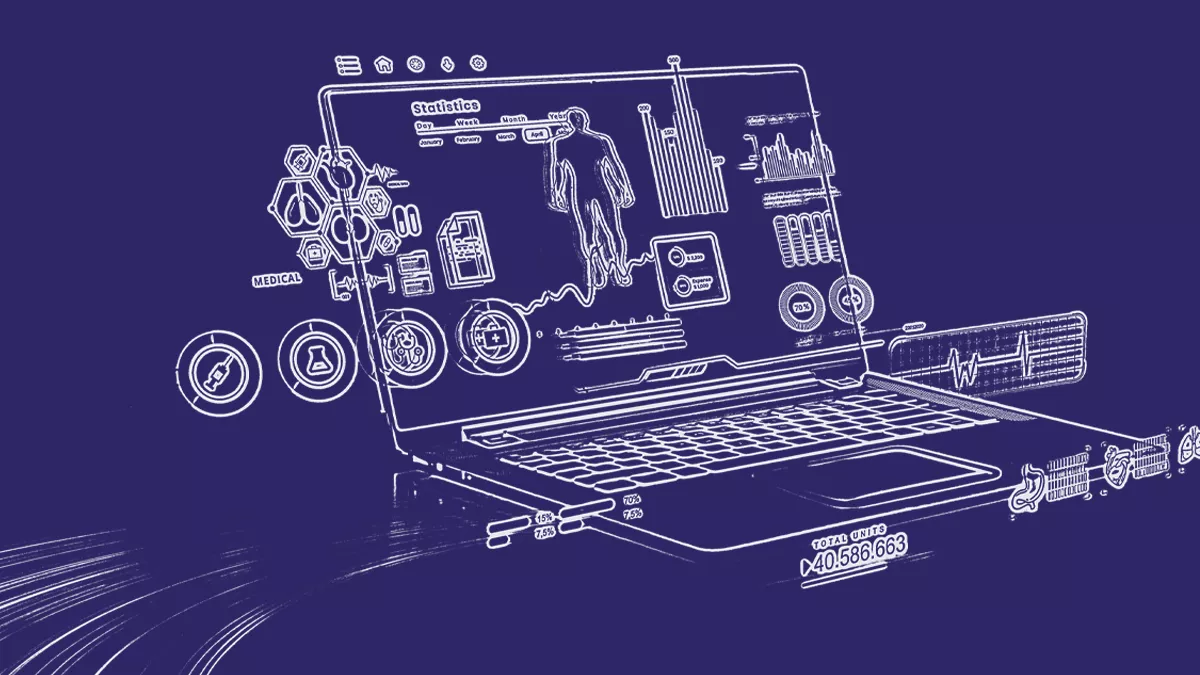
“Federated image-to-image MRI translation from heterogeneous multiple-sites data” at 2024 ISMRM & ISMRT Annual Meeting & Exhibition
There are unlikely to be many adults who have never had an MRI examination in their life. Magnetic resonance imaging or MRI is widely known as one of the most common and effective medical imaging techniques employed in medical diagnosis and treatment. Nevertheless, this technology has some shortcomings: the acquisition time of MRI data can be long, especially when multiple modalities are involved (such as T1-weighted, T2-weighted, and FLAIR). Moreover, along with multi-parameter acquisitions often come problems of missing or contradictory data.
The Translation is now regarded as a perspective Machine Learning technique, that could significantly reduce the acquisition time as we can only collect T1-weighted data and synthesize T2-weighted and FLAIR data from it, automatically solving the problem of missing data for multi-parameter acquisitions. However, Translation has its constraints, such as the need for the development of large and diverse datasets.
In a new research, Sano scientists Jan Fiszer, Dominika Ciupek, Dr. Maciej Malawski, and Dr. Tomasz Pieciak from ETSI Telecomunicación, Universidad de Valladolid, prove that Federated Learning (FL), as a method of multi-site data aggregation, is more effective for utilizing deep learning models in MRI image translation. Moreover, Federated Learning aggregates information about data from various centres without transferring the data between the sources, which lowers concerns about sharing sensitive medical data.
We are happy to share that the paper “Federated image-to-image MRI translation from heterogeneous multiple-sites data” was accepted for a poster presentation at The International Society for Magnetic Resonance in Medicine (ISMRM) & The International Society for MR Radiographers & Technologists (ISMRT) Annual Meeting & Exhibition, on 4-9 May 2024, Singapore
More about the conference https://www.ismrm.org/24m/