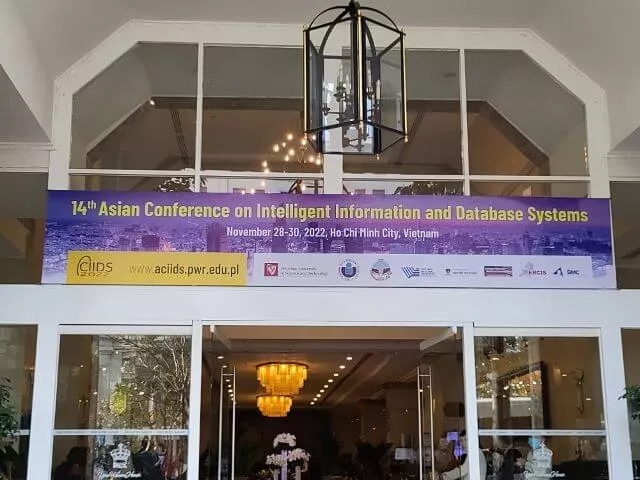
Alfredo Ibias at ACIIDS 2022 conference
From the 28th to the 30th of November Alfredo Ibias form Personal Health Data Science research team participated in the 14th Asian Conference on Intelligent Information and Database Systems (ACIIDS 2022). He presented a paper entitled “Using deep learning to detect anomalies in traffic flow”.
The aim of the conference was to provide an internationally respected forum for scientific research in the technologies and applications of intelligent information and database systems.
The conference was hosted by International University – Vietnam National University HCMC, Vietnam and jointly organized by Wrocław University of Science and Technology, Poland in cooperation with IEEE SMC Technical Committee on Computational Collective Intelligence, European Research Center for Information Systems (ERCIS), Al-Farabi Kazakh National University (Kazakhstan), University of Newcastle (Australia), Yeungnam University (Korea), Quang Binh University (Vietnam), Leiden University (The Netherlands), Universiti Teknologi Malaysia (Malaysia), Nguyen Tat Thanh University (Vietnam), BINUS University (Indonesia), Committee on Informatics of the Polish Academy of Sciences (Poland) and Vietnam National University, Hanoi (Vietnam).
The proceedings of ACIIDS 2022 will be published by Springer in series Lecture Notes in Artificial Intelligence (LNAI) and Communications in Computer and Information Science (CCIS).
In his paper, Alfredo and Manuel Mendez, and Manuel Nunez study the usefulness of two deep learning based methods (CNN auto-encoder and BiLSTM auto-encoder) to detect anomalies in situations that can be defined in terms of time series. In order to evaluate these approaches, they consider traffic flow data and perform experiments in two orthogonal scenarios: a guided scenario (training only with data considered as ’normal’ after a naive labelling) and a basic scenario. Their results show that if we train the models using only the considered ’normal’ data, the obtained models do not achieve good results because none of them are able to detect all type of abnormal data correctly. In contrast, both models can detect all type of time series anomalies when we consider the basic scenario.
The link to the publication is here.