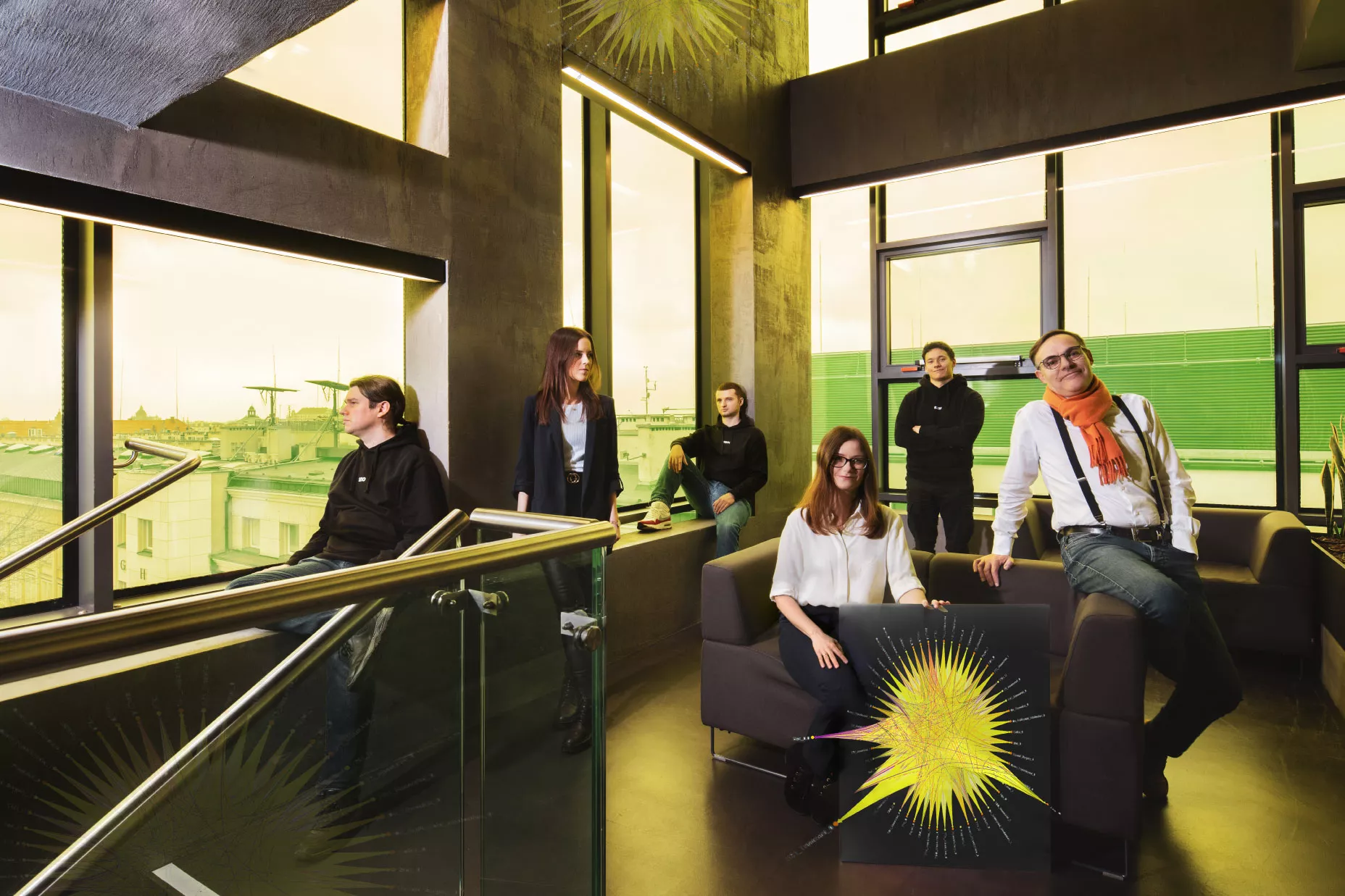
Personal Health Data Science
Behavioral science and data analytics on population health data and other types of data (e.g., wearables) to identify health risks, trends, inefficiencies, vulnerable populations, patient subgroups, and investigate potential population-wide interventions (e.g., nudge theory).