Joanna Kaleta
Endoscopic surgeries allow surgeons to operate with minimal incisions and significantly reduce patient trauma. We focus on applying deep learning to improve surgical outcomes and advance the capabilities of these procedures.
(1) Bridging the Gap between Data and Computer-Assisted Surgery:
Computer-assisted surgical systems have the potential to revolutionize surgical practices, offering surgeons real-time guidance and insights that can transform the outcomes of complex procedures. These systems rely on deep learning models that are trained on diverse and intricate datasets. However, the challenge lies in the limited availability of annotated data for training these models effectively. Generating synthetic data can overcome these limitations, but it is necessary to reduce the domain gap between real and synthetic data. We combine simulator data and generative learning methods to generate realistic fully labelled datasets.
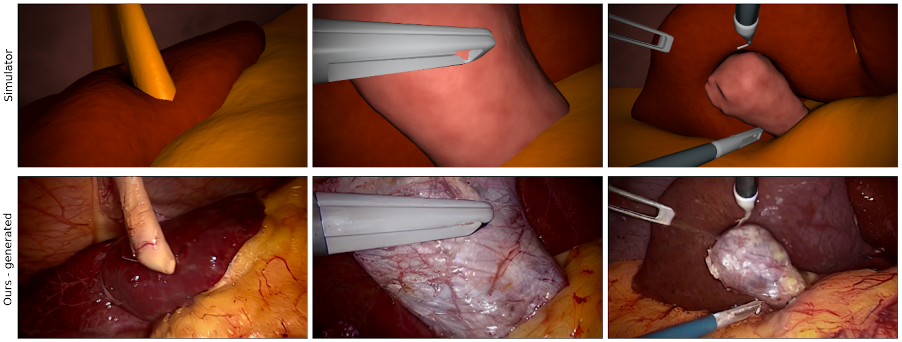
Figure 1. We utilise the Stable Diffusion model to transfer synthetic endoscopic data from a simulator into realistic images. Source: own elaboration.
(2) Advanced Visualization Tools for Fetoscopic Surgery:
Fetoscopy laser photocoagulation is a widely used procedure for the treatment of Twin-to-Twin Transfusion Syndrome (TTTS), that occurs in multiple pregnancies due to placental vascular anastomoses. We aim to develop a framework providing better visualization of the placental vessel network for TTTS treatment procedure. Our research focuses on the following areas: eliminating artefacts and enhancing the quality of endoscopic images, mosaicking, and improving placental vessel segmentation.
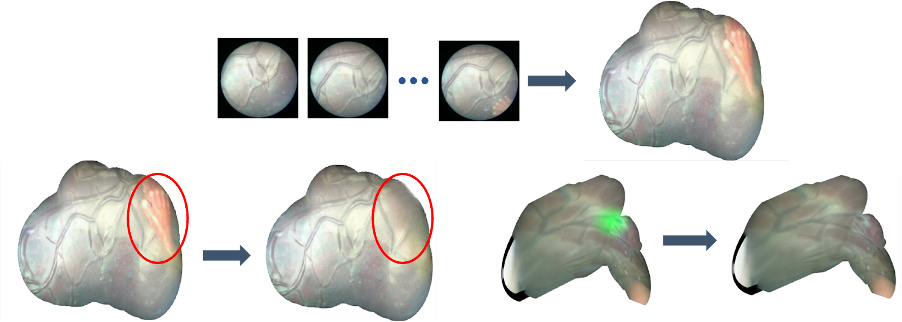
Figure 2. Example use-cases for supporting visualisation tools: mosaicking (top), inpainting and denoising (bottom). Source: Alabi et al. Robust fetoscopic mosaicking from deep learned flow fields. Int J CARS 17, 1125–1134 (2022), own elaboration.