125. Deep Learning-Based Glioma Grading and Tumor Microenvironment Characterization. Generation and Analysis of the Microscopy Image
Monika Pytlarz – PhD Student, Computer Vision (Brain&More), Sano Centre for Computational Medicine, Krakow, PL
Abstract
Gliomas, as aggressive brain tumors, necessitate precise grading for effective treatment. The role of myeloid cells in the tumor microenvironment (TME) is increasingly recognized as crucial in glioma progression and patient prognosis, highlighting the need for advanced diagnostic tools that can accurately characterize these tumors.
Current glioma grading heavily relies on manual histological evaluations, which are time-consuming, subjective, and may overlook intricate TME characteristics.
Existing glioma grading methodologies primarily employ deep learning models, such as convolutional neural networks and vision transformers, which, despite their successes, face challenges due to the need for large annotated datasets and their limited ability to capture the complex tumor microenvironment.
Our study proposes using single-cell analysis with unsupervised deep learning for the analysis of glioma single-stained tissue microarrays, focusing on the characterization of myeloid cell accumulation. The method differentiates glioma grades by identifying unique tumor microenvironment phenotypic neighborhoods.
Despite the small dataset and the challenge of distinguishing between WHO grades 2 and 3, our analysis successfully identified distinct phenotypic neighborhoods, particularly highlighting the significance of neighborhoods N2 and N4, and phenotypes P3 and P4, in differenciating malignant gliomas. This approach not only demonstrates the potential of deep learning in accurately classifying gliomas but also emphasizes the importance of myeloid cells in understanding tumor progression. Our findings suggest that automatic grading based on phenotypic neighborhoods could significantly enhance intraoperative assessments and the immunotherapies planning.
About the author
I obtained a B.Sc. in Electroradiology from the Faculty of Health Sciences of the Jagiellonian University and an M.Sc. in Bioinformatics from the Faculty of Biochemistry, Biophysics, and Biotechnology of the JU. I have experience working in a hospital’s Department of Diagnostic Imaging. My Ph.D. project focuses on the generation and analysis of microscopy images compared to other modalities.
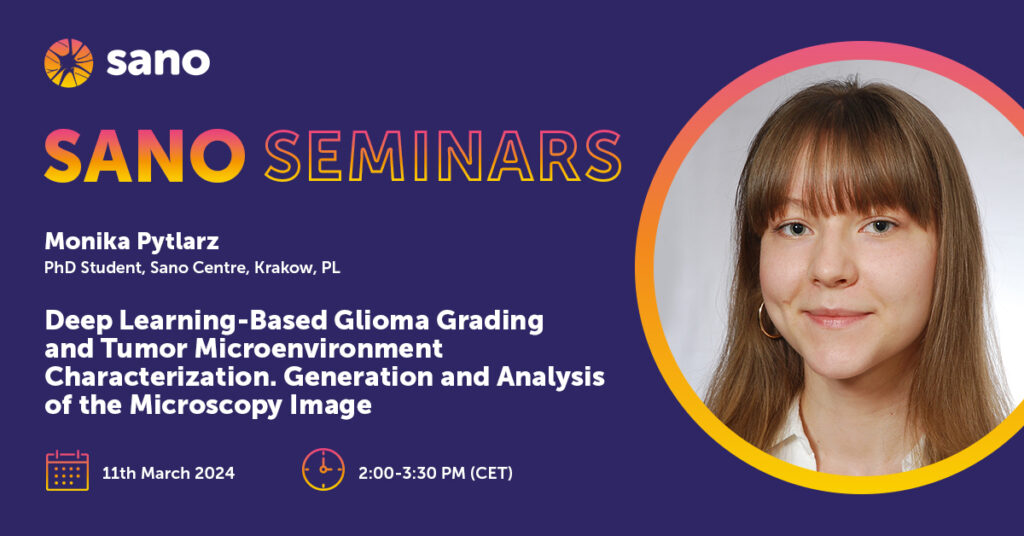