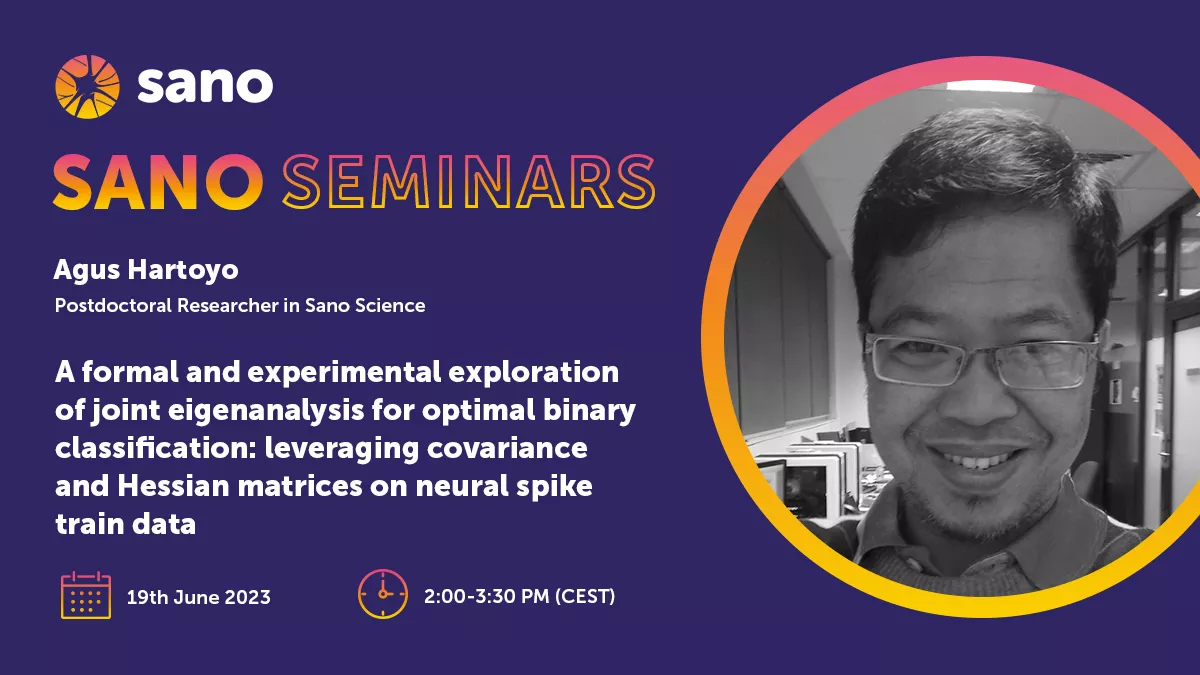
102. A formal and experimental exploration of joint eigenanalysis for optimal binary classification: leveraging covariance and Hessian matrices on neural spike train data
Agus Hartoyo – Computer Vision Data Science Team, Sano Centre for Computational Science, Krakow, PL
Abstract
The individual analysis of covariance and Hessian matrices in classification problems has been widely investigated. However, their combined potential remains largely unexplored. In this study, we propose a novel approach that integrates the eigenanalysis of a covariance matrix computed on a training set with the eigenanalysis of a Hessian matrix derived from a deep learning model. Our approach is substantiated by formal proofs that establish its capability to approximately minimize within-class variances and maximize between-class mean distance. By projecting data into the combined space of the most relevant eigendirections from both matrices, we achieve optimal class separability as per the Linear Discriminant Analysis (LDA) criteria. To validate our method, we perform extensive experiments using neural spike train data, supporting its efficacy through empirical evidence. Our experimental results demonstrate superior performance compared to UMAP in terms of generalization and surpass LDA in terms of dimensionality. Furthermore, the analysis of the spike train data reveals the crucial role of capturing differences or changes in activity, highlighting the significance of this parameter over frequency-based characteristics. Leveraging the computation of the Hessian matrix from a deep learning model, our approach provides insights into the underlying mechanisms of black-box models. This comprehensive investigation establishes a new avenue for interpreting binary classifiers and holds promise for extending its applicability to multi-class problems.
About the author
Agus is a computational neuroscientist with a Ph.D. in computational neuroscience from Swinburne University of Technology, Australia. Prior to his Ph.D., he obtained an M.Sc. in Computer Science from TU Kaiserslautern, Germany. Agus has a keen interest in computational neuroscience, statistical inference, machine learning, logic, and formal methods. Currently, he serves as a postdoctoral researcher at Sano, where he explores neural spike train classification and investigates the comorbidity of PTSD and other disorders through machine learning analysis of EEG data.