156. Augmenting MRI scan data with real-time predictions
Maciej Paszyński, Faculty of Computer Science, AGH University
Abstract:
Simulations of tumor growth rely on mathematical models describing the tumor evolution and efficient and accurate numerical solvers performing 3D computations, including spatial and temporal discretizations. The finite difference 3D tumor growth solver [1] based on advection–diffusion–reaction equations, can solve one time step on 250 × 250 × 250 stencil points using one GPU within 100 sec. The higher-order finite element method solver takes 2122s on CPU or 12 sec on two GPUs to compute a one-time step on 256 × 256 × 256 mesh using isogeometric finite element method solver [2]. Summing up, efficient tumor simulators require parallel computations. In this talk, we present a novel computational method, the exponential integrators, that replaces the time integration scheme with integral equations that can be solved efficiently on a laptop. It can be applied to large class of PDEs and different spatial discretizations. In our presentation we focus on the glioblastoma brain tumor modeled with the Fisher–Kolmogorov diffusion–reaction model with logistic growth. We present a MATLAB simulator that can compute one time steps on 128 x 128 x 128 finite difference stencil within 7 sec on a laptop with single CPU. The input is the MRI scans of the human head and the initial tumor location. The simulation uses the finite difference formulation in space and the ultra-fast exponential integrators method in time. The output from the code is the input data for ParaView visualization. While there are many brain tumor simulation codes, our method’s novelty lies in its implementation using exponential integrators. We propose a new algorithm for the fast computation of exponential integrators [3]. Regarding execution time on a laptop with Win10, using MATLAB, with 11th Gen Intel(R) Core(TM) i5-11500H, 2.92 GHz, and 32 GB of RAM, the algorithm outperforms the state-of-the-art routines from Al-Mohy and Higham [4]. We also compare our method with an implicit, unconditionally stable Crank–Nicolson time integration scheme based on the finite difference method. We show that our method is two orders of magnitude faster than the Crank–Nicolson method with finite difference discretization in space on a laptop equipped with MATLAB. The brain tumor’s two-year future prediction using a computational grid and 100-time steps, built over the MRI scans of the human head, takes less than 10 minutes on the laptop.
[1] A. Kłusek, M. Łoś, M. Paszyński, W. Dzwinel, Efficient model of tumor dynamics simulated in multi-GPU environment, International Journal of High Performance Computer Applications, 33 (2019), 489-506
[2] L. Siwik, M. Łoś, A. Kłusek, A. Paszyńska, K. Pingali, W. Dzwinel, M. Paszyński, Tuning three-dimensional tumor progression simulations on a cluster of GPGPUs, Journal of Computational and Applied Mathematics, 412 (2022), Article 114308
[3] M. Pabisz, J. Munoz-Matute, M. Paszyński, Augmenting MRI scan data with real-time predictions of glioblastoma brain tumor evolution using faster exponential time integrators, Journal of Computational Science, 85 (2025), Article 102493
[4] A. Al-Mohy, N. Higham, Computing the action of the matrix exponential, with an application to exponential integrators, SIAM Journal of Scientiffic Computing, 33 (2011) 488-511
About the author:
Maciej Paszyński, Faculty of Computer Science, AGH University
Full Professor of Computer Science
Ph.D. in Mathematics with Applications to Computer Science
h-index: 23 (Web of Science) 22 (Scopus) 28 (Google Scholar)
He leads the group “Adaptive Algorithms and Systems (A2S)” working in the area of applications of artificial intelligence (AI) and high-performance computing (HPC) in advanced simulations.
https://home.agh.edu.pl/~paszynsk/
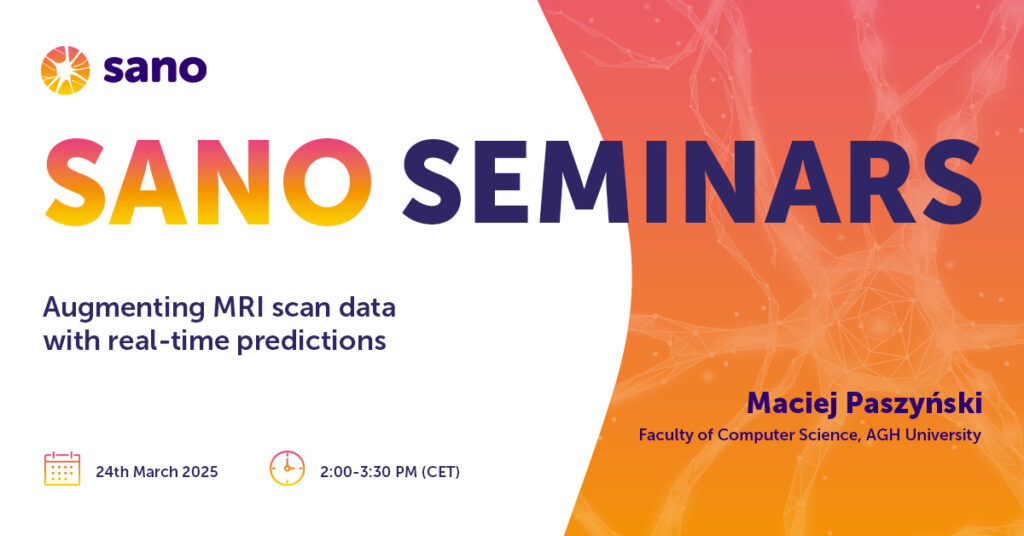