134. Multi-modal hybrid approach to data mining for medical diagnosis
Kamil Wozniak – PhD Student, Personal Health Data Science, Sano Centre for Computational Medicine, Krakow, PL
Abstract
On one hand, deep learning-based methods have taken the AI field by storm [1], as they provide unmatched performance in many tasks but require a lot of data and computational resources while decisions made by them are often far from transparent and interpretable by humans [2,3]. On the other hand, classical approaches offer methods that do not achieve state-of-the-art performance but are less resource-demanding and provide easily interpretable results. Transparency and interpretability are essential in the medical field, where error costs can be very high. Medical data also comes in many different and inconsistent types making an overall analysis of it challenging [5]. Moreover, interpretable rule-based solutions have previously produced satisfactory results while maintaining high prediction quality [4]. Here, the idea of combining different types of data, as well as new deep learning models with knowledge mining and logic programming is presented. This allows higher-level abstract representations of the data to be built, thereby allowing for quick, explainable diagnostic decisions. It builds on CACTUS [6], a classification tool developed by the team, and this work is part of a larger research effort of the whole team to create continuously learning solution.
[1] Dean, Jeffrey. “A Golden Decade of Deep Learning: Computing Systems & Applications.” Daedalus 151 (2022): 58-74.
[2] Yang, Sijie et al. “Intelligent Health Care: Applications of Deep Learning in Computational Medicine.” Frontiers in Genetics 12 (2021).
[3] Hayashi, Yoichi. “Black Box Nature of Deep Learning for Digital Pathology: Beyond Quantitative to Qualitative Algorithmic Performances.” AI and ML for Digital Pathology (2020).
[4] Bajcar, S., Grzymala-Busse, J.W., Grzymala-Busse, W.J., & Hippe, Z.S. (2003). Diagnosis of Melanoma Based on Data Mining and ABCD Formulars. International Conference on Health Information Science.
[5] Aldoseri, Abdulaziz et al. “Re-Thinking Data Strategy and Integration for Artificial Intelligence: Concepts, Opportunities, and Challenges.” Applied Sciences (2023): n. pag.
[6] Gherardini, L., Varma, V. R., Capała, K., Woods, R. & Sousa, J. CACTUS: a Comprehensive Abstraction and Classification Tool for Uncovering Structures. ACM Trans. Intell. Syst. Technol. 15, 1–23 (2024).
About the author
Kamil has a master’s degree in computer science and at Sano he continues his development in this field as a PhD student, where he works on combining statistical and symbolic approaches to artificial intelligence and its application to biomedical data. He is also interested in integrating data of various types (including laboratory measurements, imaging and written text) and combining them into higher-level abstractions.
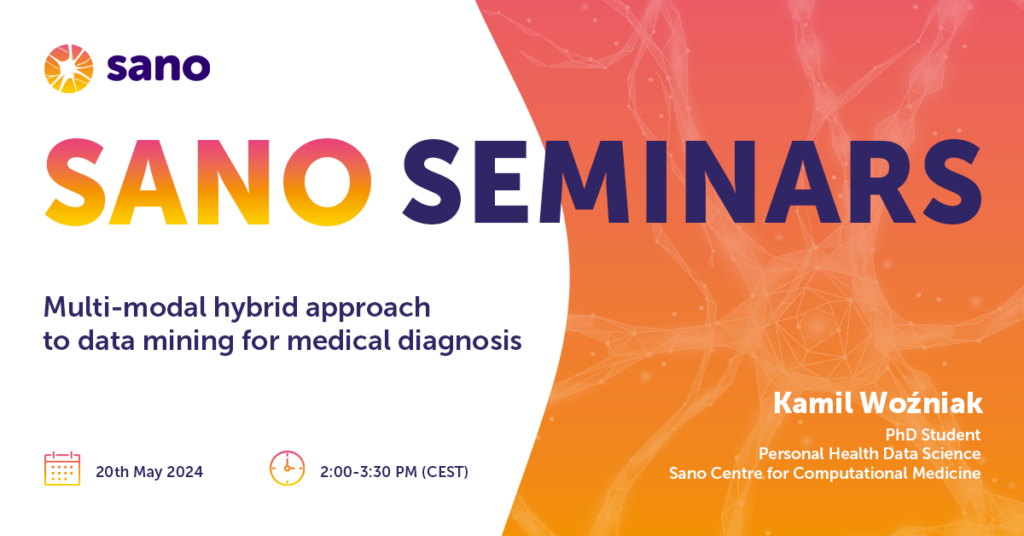