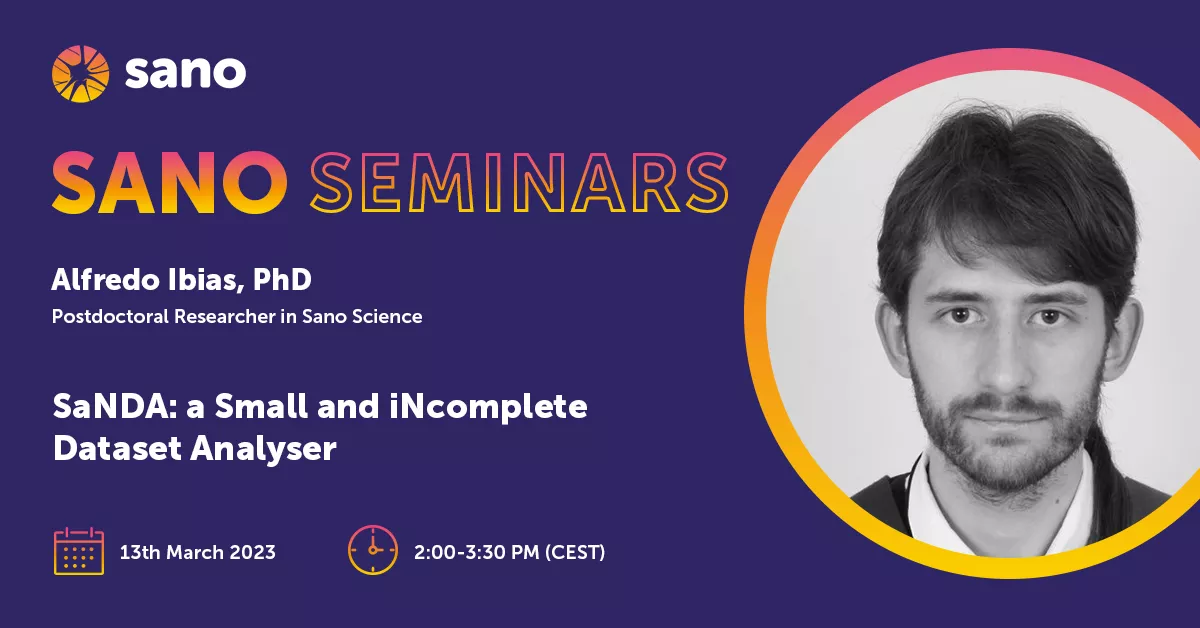
91. SaNDA: a Small and iNcomplete Dataset Analyser
Alfredo Ibias, Personal Health Data Science Team, Sano Centre for Computational Medicine, Krakow, PL
Abstract
In personalised health, small datasets with missing data are quite common. Current Machine Learning methods are unable to process such datasets in a meaningful way due to the huge data volume requirement. To address this problem, we proposed a new Small and iNcomplete Dataset Analyser (SaNDA) to process such datasets in a meaningful way. Due to the characteristics of these datasets and the criticality of the domain, an explainable method was mandatory. Thus, SaNDA prioritised explainability over efficiency. We evaluated our proposal against the current standard of explainable methods: Random Forests. We observed that our proposal outperforms Random Forest when there is more missing data and/or lower number of entries in the dataset, obtaining less favourable results over typically larger, well-curated datasets. Given the difficulties in obtaining complete, reliable data in the healthcare field, we consider that our proposal could be useful for practitioners.
About the author
Alfredo Ibias received B.A. degrees in Computer Science and in Mathematics from Complutense University of Madrid, Spain; an M.A. degree in Formal Methods in Computer Science from the same university; and a Ph.D. degree in Computer Science at the same university too. He is currently working at Sano as a Postdoctoral Researcher focused on developing AI methods for healthcare.