113. Time-based clustering and visual data analytics of longitudinal healthcare data
Dr Maria-Cruz Villa-Uriol – Senior Lecturer INSIGNEO Institute for in silico medicine, Healthy Lifespan Institute, Dept Computer Science, University of Sheffield, UK
Abstract
Our society is witnessing an exponential growth of data being generated and collected. Despite historical and structural digitalisation challenges, healthcare is an example where the analysis of longitudinal data might bring a new revolution.
In this talk, I will present our recent efforts in analysing and exploring longitudinal healthcare data. First, we have developed a visual analytics approach able to summarise and seamlessly explore large volumes of complex event data sequences. We are able to easily derive observations and findings that otherwise would have required a significant investment of time and effort. To facilitate the identification of findings, we use a hierarchical clustering approach to cluster sequences according to time and a novel visualisation environment. To show the benefits of this approach, I will present our results in one real case study where we have analysed the calls and responses of emergency services to identify bottlenecks and inefficiencies.
And second, I will share our most recent work in the analysis of clinical events extracted from Electronic Health Records for the study of multimorbidity. I will present a machine learning pipeline able to cluster longitudinal disease sequences at multiple levels of detail. Our method uses a two-stage approach using Hidden-Markov Models to account for time and graph theory to identify groups of relevant solutions. I will conclude by focusing on how to interpret and validate the many alternative clustering results offered by these techniques, and the implications that their use has on the study of multimorbidity using longitudinal healthcare datasets.
About the author
Dr Maria-Cruz Villa-Uriol is a Senior Lecturer in the Department of Computer Science at the University of Sheffield. She is a member of the INSIGNEO Institute for in silico Medicine, and the Healthy Lifespan Institute (HELSI). She combines visualisation and machine learning to support clinical decision making for heterogeneous data sources such as healthcare operational data, electronic patient health records and in silico models.
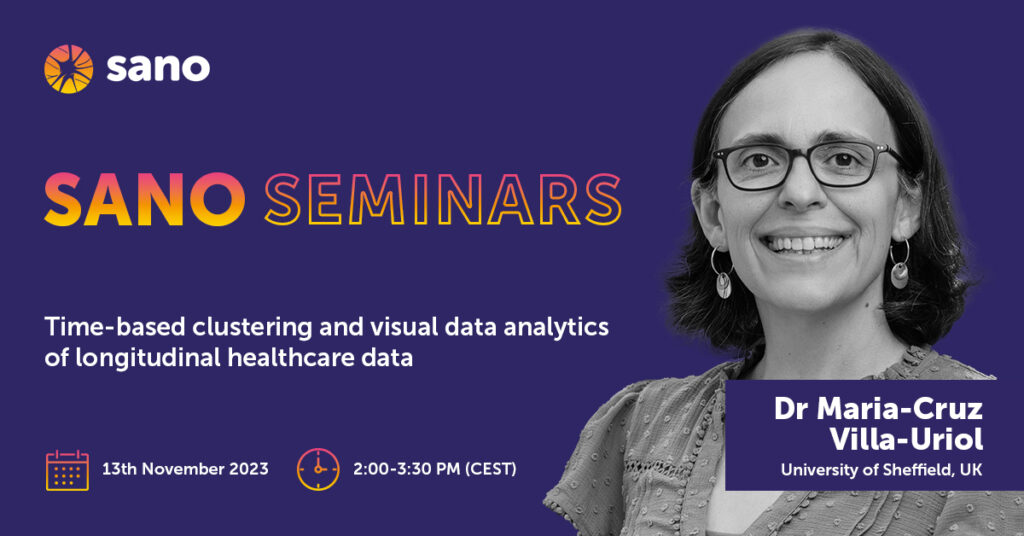