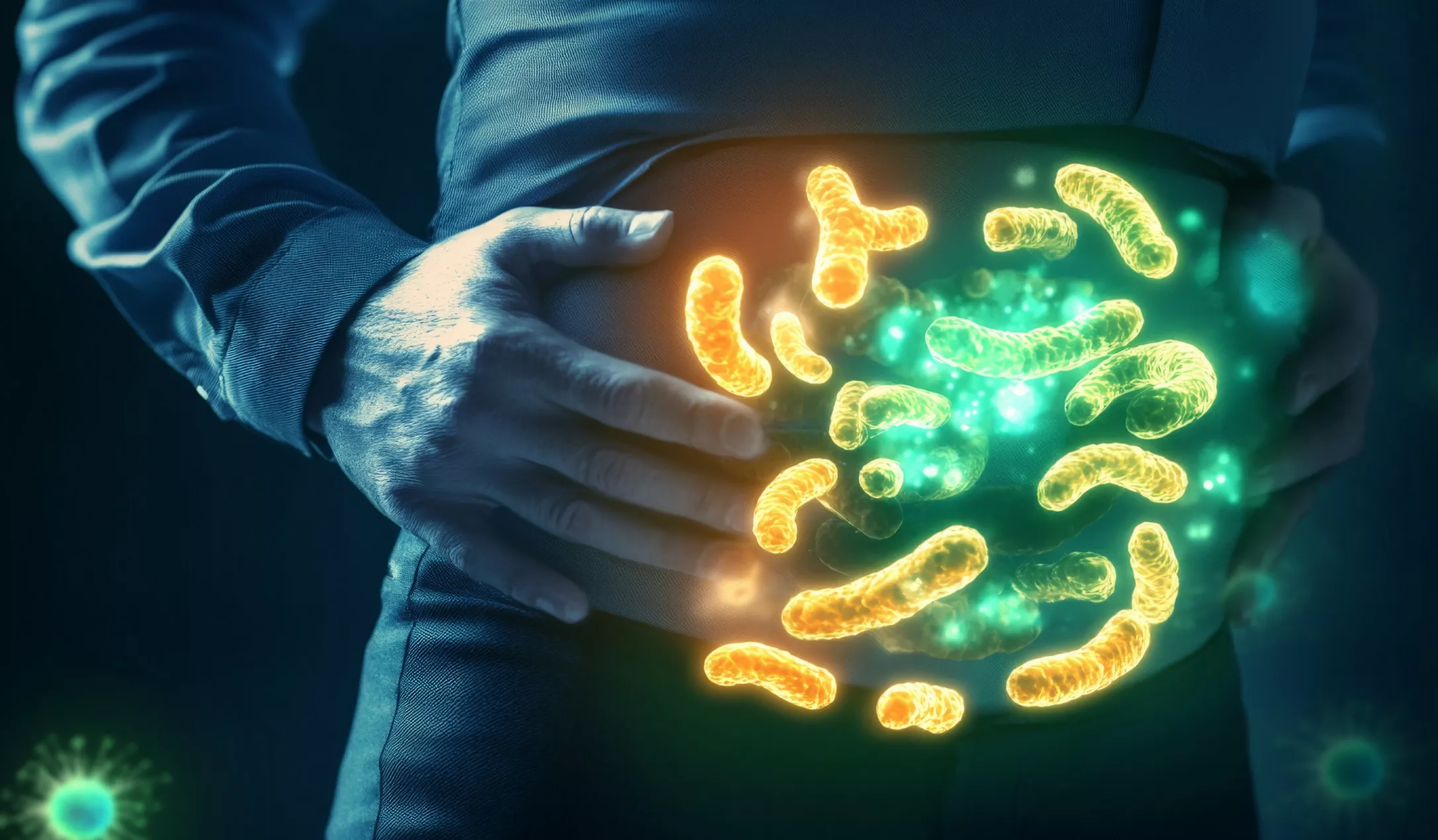
Study by Polish-Estonian team establishes guidelines for better use of microbiome data in disease diagnostic
As many as 100 trillion microorganisms live in the human gut, forming a unique microbiome. The microbiome is constantly supporting your health – helping with digestion, producing vitamins, boosting immunity and even influencing your mood. Imagine if we could determine disease occurrence and risk of developing a disease based on the microbiome composition. Developing methods that enable such analysis is the future of medicine – the key to innovative ways of diagnosis and treatment. Groundbreaking research by a team from the Sano – Center for Computational Medicine, the Jagiellonian University’s Malopolska Biotechnology Center and the University of Tartu opens the door to this fascinating but still little understood world.
Can the choice of microbiome analysis method change the accuracy of disease diagnostics and conclusions about the role of microorganisms in disease pathophysiology? Scientists have proven that it does. The findings have just been published in the prestigious journal Microbiome, opening up new possibilities for future research.
The way we analyze the microbiome – the invisible communities of bacteria that inhabit our intestines – has a huge impact on identifying key microorganisms for a given disease. These are the ones that can be both guardians of health and harbingers of disease. The study’s authors emphasize that standardization of analytical methods is not only a necessity, but actually the foundation for future discoveries.
The authors employed machine learning algorithms and various data transformation techniques to analyze over 8,500 samples from 24 distinct datasets. Their aim was to enhance the accuracy of differencing microbial profiles between diseased and healthy states, while also gaining deeper insights into how the choice of method influences the identification of key bacteria.
The publication is also a step toward using the microbiome as a tool for creating personalized therapies and accurate diagnostics.
The gut microbiome, and a breakthrough in disease diagnosis
Traditional diagnostic methods for certain diseases can require expensive and invasive testing, but studies of the gut microbiota show that changes in its composition can act as natural biomarkers.
For example, specific patterns in the microbiome – an increased abundance of some bacteria or a lack of others – can indicate the onset of serious conditions before a patient even notices any symptoms. It’s like an early warning system that could detect health problems before any symptoms appear. Colorectal and pancreatic cancer are notable examples where the microbiome promises to improve disease diagnostics.
What’s more, methods based on microbiome diagnostics are less invasive and burdensome for patients, offering a new quality in preventive medicine.
Machine learning in microbiome analysis
Artificial intelligence brings a new quality to microbiome analysis. It can handle the enormous complexity and diversity of biological data. Machine learning-based technologies allow more precise detection of disease-associated patterns and interpretation of data, opening up opportunities for clinical breakthroughs.
Transforming raw microbiome data into understandable and analyzable forms is a key step in microbial research. However, the application of these transformations in combination with machine learning is still poorly explored.
Experts point to an urgent need to standardize analysis methods, which, combined with the power of artificial intelligence, could change the way the microbiome is understood and used in medicine.
Simple techniques equally effective
The authors of the cited paper used machine learning algorithms and data transformation to analyze more than 8,500 samples from 24 different datasets to improve classification accuracy and better understand how method selection affects the identification of important bacteria. Their results suggest that it is possible to distinguish between healthy and sick people based on microbiome data. They found that the results of the analysis depended little on which algorithm or data transformation method was used. The study showed that simple analysis techniques, like assessing the presence of specific bacteria, can be just as effective as more complex methods. A small set of bacteria is sufficient for diagnosis, but different analysis techniques point to different key bacteria.
The results of this study could dramatically change the way we think about disease, showing that the bacteria in our guts know more about us than we do ourselves. It highlights the importance of the way we analyze microbiome data, especially given the rapid expansion of microbiome datasets. As machine learning methods continue to evolve, they will be instrumental in understanding microbiome changes and their complex relationship to human health. This approach will be pivotal in shaping future advancements in personalized medicine and disease prevention. This is the future of medicine, which is just starting to gain momentum.
Autors: Zuzanna Karwowska, Oliver Aasmets